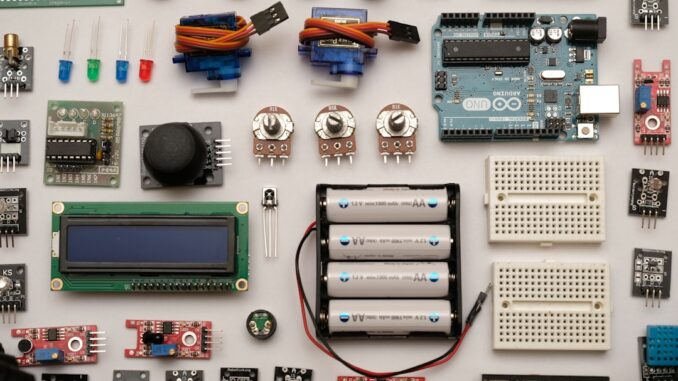
Abstract
This research report explores the multifaceted domain of maintenance, moving beyond simplistic notions of repair to examine proactive and predictive strategies for enhancing system resilience across diverse applications. Departing from a focus solely on home maintenance, the report adopts a broader, multi-disciplinary perspective, drawing insights from engineering, operations management, data science, and materials science. We investigate various maintenance paradigms, including preventive maintenance, condition-based maintenance, and predictive maintenance, analyzing their strengths and weaknesses in different contexts. Furthermore, the report delves into advanced techniques such as machine learning for predictive maintenance, the role of sensor technologies and the Internet of Things (IoT) in condition monitoring, and the optimization of maintenance schedules using mathematical modeling. The objective is to provide a comprehensive overview of the evolving landscape of maintenance practices, emphasizing the shift towards data-driven and proactive approaches to minimize downtime, reduce costs, and improve the overall performance and lifespan of complex systems. The report concludes with a discussion of future research directions and the challenges associated with implementing advanced maintenance strategies in real-world scenarios.
Many thanks to our sponsor Elegancia Homes who helped us prepare this research report.
1. Introduction
Maintenance, historically viewed as a reactive response to system failures, has evolved into a proactive discipline aimed at preventing failures and maximizing system availability. This shift is driven by increasing complexity in engineered systems, stringent performance requirements, and the economic imperative to minimize downtime and operational costs. While the concept of maintenance is universally applicable, its specific implementation varies significantly across industries, ranging from manufacturing and transportation to healthcare and infrastructure. A deeper understanding of maintenance principles and their application is crucial for engineers, managers, and policymakers seeking to optimize system performance and ensure long-term sustainability.
This report adopts a multi-disciplinary approach to explore the current state-of-the-art in maintenance strategies. We move beyond basic repair and replacement activities to examine the broader context of system lifecycle management, focusing on proactive techniques that leverage data analytics, sensor technologies, and advanced modeling to anticipate and prevent failures. The report will examine, compare and contrast various approaches.
Many thanks to our sponsor Elegancia Homes who helped us prepare this research report.
2. Maintenance Paradigms: A Comparative Analysis
Several distinct maintenance paradigms have emerged, each with its own set of principles, methodologies, and applications. Understanding these paradigms is essential for selecting the most appropriate maintenance strategy for a given system or application.
2.1 Reactive Maintenance
Reactive maintenance, also known as breakdown maintenance, is the most basic approach, involving repairs only after a failure has occurred. This strategy is typically adopted for non-critical systems where the cost of failure is low, and the implementation of proactive maintenance measures is not economically justified. While reactive maintenance may appear to be the simplest option, it can lead to unexpected downtime, increased repair costs, and potential safety hazards. Furthermore, it provides no opportunity to learn from past failures or to improve system reliability.
2.2 Preventive Maintenance (PM)
Preventive maintenance (PM) involves performing maintenance tasks at predetermined intervals, regardless of the actual condition of the equipment. These tasks may include inspections, lubrication, cleaning, and component replacement. PM is based on the assumption that equipment deteriorates over time and that regular maintenance can prevent failures. While PM can be effective in reducing the frequency of failures, it can also be inefficient, as it may lead to unnecessary maintenance activities and the replacement of components that are still in good condition. The effectiveness of PM depends heavily on the accuracy of the maintenance schedule, which should be based on historical data, manufacturer recommendations, and operational experience. A key disadvantage is that PM schedules do not take account of specific working conditions and load.
2.3 Condition-Based Maintenance (CBM)
Condition-based maintenance (CBM) relies on monitoring the condition of equipment and performing maintenance only when there is evidence of deterioration or an impending failure. CBM utilizes a variety of sensors and diagnostic techniques to assess the health of equipment, including vibration analysis, oil analysis, thermography, and ultrasonic testing. The advantages of CBM include reduced maintenance costs, extended equipment lifespan, and improved system reliability. However, CBM requires a significant investment in sensors, data acquisition systems, and diagnostic expertise. The success of CBM also depends on the ability to accurately interpret the data and to identify potential problems before they lead to failures. A key problem can be with the accuracy of sensors, sensor failure, or the cost of installing sensors on legacy equipment.
2.4 Predictive Maintenance (PdM)
Predictive maintenance (PdM) goes a step further than CBM by using advanced data analytics and machine learning techniques to predict future failures and to optimize maintenance schedules. PdM leverages historical data, real-time sensor data, and mathematical models to identify patterns and trends that indicate potential problems. The advantages of PdM include minimizing downtime, reducing maintenance costs, and improving system performance. However, PdM requires a significant investment in data infrastructure, analytical tools, and data science expertise. The accuracy of PdM depends heavily on the quality and completeness of the data, as well as the sophistication of the analytical models. It also requires understanding the limitations of the models and implementing robust validation procedures. Data governance is also vital as data collection and security have to be closely monitored.
2.5 Reliability-Centered Maintenance (RCM)
Reliability-centered maintenance (RCM) is a systematic approach to maintenance planning that focuses on identifying the critical functions of a system and developing maintenance strategies to ensure that those functions are maintained at a specified level of reliability. RCM involves a detailed analysis of potential failure modes, their causes, and their consequences. Based on this analysis, maintenance tasks are selected that are most effective in preventing failures or mitigating their consequences. RCM is a comprehensive and rigorous approach that can lead to significant improvements in system reliability and reduced maintenance costs. However, RCM requires a significant investment in engineering resources and a thorough understanding of system behavior.
Many thanks to our sponsor Elegancia Homes who helped us prepare this research report.
3. Advanced Techniques in Predictive Maintenance
Predictive maintenance (PdM) has emerged as a powerful tool for optimizing maintenance schedules and minimizing downtime. PdM leverages advanced data analytics and machine learning techniques to predict future failures and to proactively address potential problems. Some of the key techniques used in PdM include:
3.1 Machine Learning for Failure Prediction
Machine learning (ML) algorithms can be trained on historical data to identify patterns and trends that indicate potential failures. Supervised learning algorithms, such as classification and regression models, can be used to predict the probability of failure or the remaining useful life of equipment. Unsupervised learning algorithms, such as clustering and anomaly detection, can be used to identify unusual patterns in the data that may indicate a developing problem. The performance of ML models depends heavily on the quality and completeness of the data, as well as the selection of appropriate features and algorithms. Data pre-processing, feature engineering, and model validation are crucial steps in the development of effective ML-based PdM systems. It is vital to ensure that machine learning models are explainable to users as there is a risk that their predictions will not be used if they are percieved as a ‘black box’.
3.2 Sensor Technologies and the Internet of Things (IoT)
The Internet of Things (IoT) has enabled the widespread deployment of sensors to monitor the condition of equipment in real-time. These sensors can measure a variety of parameters, such as vibration, temperature, pressure, and flow rate. The data collected by these sensors can be transmitted wirelessly to a central data repository for analysis. IoT-enabled PdM systems offer several advantages, including continuous monitoring, early detection of potential problems, and remote access to data. However, the implementation of IoT-based PdM systems requires careful consideration of sensor selection, data acquisition systems, communication protocols, and data security. Issues with data transmission and management will often be seen when applying this approach.
3.3 Data Analytics and Signal Processing
Data analytics plays a crucial role in PdM by transforming raw sensor data into actionable insights. Signal processing techniques can be used to extract relevant features from the data, such as frequency components, amplitudes, and statistical measures. These features can then be used as inputs to ML models or to develop rule-based diagnostic algorithms. Data visualization techniques can be used to present the results of the analysis in a clear and concise manner. The choice of appropriate data analytics techniques depends on the type of data being collected, the nature of the potential failures, and the desired level of accuracy.
3.4 Digital Twins and Simulation
Digital twins are virtual representations of physical assets or systems that can be used to simulate their behavior under different operating conditions. Digital twins can be used to test different maintenance strategies, to predict the impact of failures, and to optimize system performance. The development of accurate digital twins requires a deep understanding of the physical system, as well as the ability to integrate data from various sources, such as sensors, historical records, and engineering models. Digital twins offer a powerful tool for PdM, but their implementation requires significant investment in modeling, simulation, and data integration capabilities.
Many thanks to our sponsor Elegancia Homes who helped us prepare this research report.
4. Optimization of Maintenance Schedules
Optimizing maintenance schedules is a critical aspect of effective maintenance management. The goal of optimization is to minimize the total cost of maintenance while ensuring that system reliability remains at an acceptable level. Several techniques can be used to optimize maintenance schedules, including:
4.1 Mathematical Modeling and Optimization Algorithms
Mathematical models can be used to represent the degradation process of equipment and the impact of maintenance activities. These models can then be used to optimize maintenance schedules by minimizing the total cost of maintenance, subject to constraints on system reliability, availability, and safety. Optimization algorithms, such as linear programming, dynamic programming, and genetic algorithms, can be used to solve these models. The accuracy of the models and the efficiency of the algorithms are crucial for obtaining optimal maintenance schedules. Care must be taken in ensuring the optimisation constraints are realistic and representative of the real world.
4.2 Risk-Based Maintenance (RBM)
Risk-based maintenance (RBM) prioritizes maintenance activities based on the risk associated with potential failures. Risk is defined as the product of the probability of failure and the consequence of failure. RBM involves identifying the critical components of a system, assessing the probability of failure for each component, and estimating the consequence of failure. Maintenance activities are then prioritized based on the risk associated with each component. RBM can be used to allocate maintenance resources more effectively and to focus on the components that pose the greatest risk to system performance. It will be difficult to apply RBM without accurate data on failure modes and rate.
4.3 Condition Monitoring and Adaptive Scheduling
Condition monitoring can be used to adjust maintenance schedules based on the actual condition of the equipment. When the condition of the equipment is good, the maintenance interval can be extended. When the condition of the equipment is deteriorating, the maintenance interval can be shortened. Adaptive scheduling can be implemented using rule-based systems or machine learning algorithms. The effectiveness of adaptive scheduling depends on the accuracy of the condition monitoring data and the responsiveness of the scheduling system.
Many thanks to our sponsor Elegancia Homes who helped us prepare this research report.
5. Challenges and Future Directions
While proactive maintenance strategies offer significant benefits, their implementation is not without challenges. Some of the key challenges include:
5.1 Data Quality and Availability
The effectiveness of PdM and CBM depends heavily on the availability of high-quality data. However, in many organizations, data is fragmented, incomplete, or inaccurate. Improving data quality and availability requires a comprehensive data management strategy that includes data collection, storage, cleaning, and validation. Data governance policies and procedures are also essential to ensure the integrity and security of the data. The cost of acquiring the data must also be considered.
5.2 Integration with Existing Systems
Integrating advanced maintenance strategies with existing systems can be complex and costly. Legacy systems may not be compatible with new technologies, and data integration may require significant customization. A phased approach to implementation is often recommended, starting with pilot projects and gradually expanding to other areas of the organization. This will allow the organization to demonstrate the benefits of proactive maintenance and to build the necessary expertise. Ensuring integration with legacy systems can often be a significant obstacle.
5.3 Skill Gaps and Training
The implementation of advanced maintenance strategies requires a skilled workforce with expertise in data analytics, machine learning, and sensor technologies. However, many organizations face a shortage of qualified personnel in these areas. Training programs and partnerships with universities and technical schools can help to bridge the skill gap. It is also important to foster a culture of continuous learning and to encourage employees to develop their skills. The skills are not just technical but also involve understanding the operation of the systems that are being monitored.
5.4 Cost Justification and Return on Investment (ROI)
Justifying the investment in proactive maintenance strategies can be challenging, particularly in the short term. It is important to develop a clear understanding of the potential benefits of proactive maintenance, such as reduced downtime, lower maintenance costs, and improved system performance. A detailed cost-benefit analysis should be conducted to demonstrate the ROI of the investment. It is also important to track the results of the implementation and to communicate the benefits to stakeholders. It may be necessary to adopt a staged implementation to allow benefits to be quantified before full deployment. The ROI can often be seen in risk reduction which is notoriously difficult to measure.
Future research directions in maintenance include:
- Development of more robust and explainable machine learning models for failure prediction.
- Integration of physics-based models with data-driven models to improve the accuracy of predictions.
- Development of new sensor technologies for condition monitoring.
- Application of blockchain technology for secure data sharing and provenance.
- Development of autonomous maintenance systems that can perform repairs and replacements without human intervention.
Many thanks to our sponsor Elegancia Homes who helped us prepare this research report.
6. Conclusion
Maintenance has evolved from a reactive to a proactive discipline, driven by the increasing complexity of engineered systems and the economic imperative to minimize downtime and operational costs. Proactive maintenance strategies, such as preventive maintenance, condition-based maintenance, and predictive maintenance, offer significant benefits in terms of reduced downtime, lower maintenance costs, and improved system performance. The implementation of these strategies requires a multi-disciplinary approach, leveraging data analytics, sensor technologies, and advanced modeling. While challenges remain in terms of data quality, integration with existing systems, and skill gaps, the potential benefits of proactive maintenance are significant. Future research directions include the development of more robust machine learning models, the integration of physics-based models with data-driven models, and the development of autonomous maintenance systems. By embracing proactive maintenance strategies, organizations can enhance system resilience, improve operational efficiency, and ensure long-term sustainability.
Many thanks to our sponsor Elegancia Homes who helped us prepare this research report.
References
- Ahmad, R., & Kamaruddin, S. (2012). An overview of time-based and condition-based maintenance in industrial application. Computers & Industrial Engineering, 63(1), 135-149.
- Jardine, A. K. S., Lin, D., & Banjevic, D. (2006). A review on machinery diagnostics and prognostics implementing condition-based maintenance. Mechanical Systems and Signal Processing, 20(7), 1483-1510.
- Lee, J., Lapira, E., Bagheri, B., & Kao, H. A. (2013). Recent advances and trends in predictive manufacturing systems in the era of industry 4.0. Manufacturing Letters, 1(1), 6-9.
- Mobley, R. K. (2002). An introduction to predictive maintenance. Butterworth-Heinemann.
- Prajapati, D., Kant, R., & Shankar, R. (2012). A fuzzy AHP and DEA integrated approach for evaluating performance of third party reverse logistics service providers. International Journal of Production Economics, 135(2), 540-552.
- Si, X. S., Wang, W., Hu, C. H., & Zhou, D. H. (2011). Remaining useful life prediction—A review on the statistical data driven approaches. European Journal of Operational Research, 213(1), 1-14.
- Vogl, G. W., Weiss, B. A., & Helu, M. (2016). A review of machine-learning approaches for predictive maintenance. Procedia CIRP, 52, 27-32.
Be the first to comment